Governance, Quality Control, and Ethical Oversight in AI
In today’s fast-paced digital landscape, AI Governance is crucial for ensuring that AI systems are ethical, secure, and trustworthy. As Artificial Intelligence (AI) continues to reshape industries, responsible governance ensures that these technologies are fair and free from bias.
This is where governance, quality control, and ethical oversight come into play. Managing risks and ensuring accountability across each stage of the AI model lifecycle is essential for fostering trust in AI systems. By adopting strong governance measures and applying rigorous quality control, organisations can build AI solutions that are not only powerful but also ethical, fair, and secure.
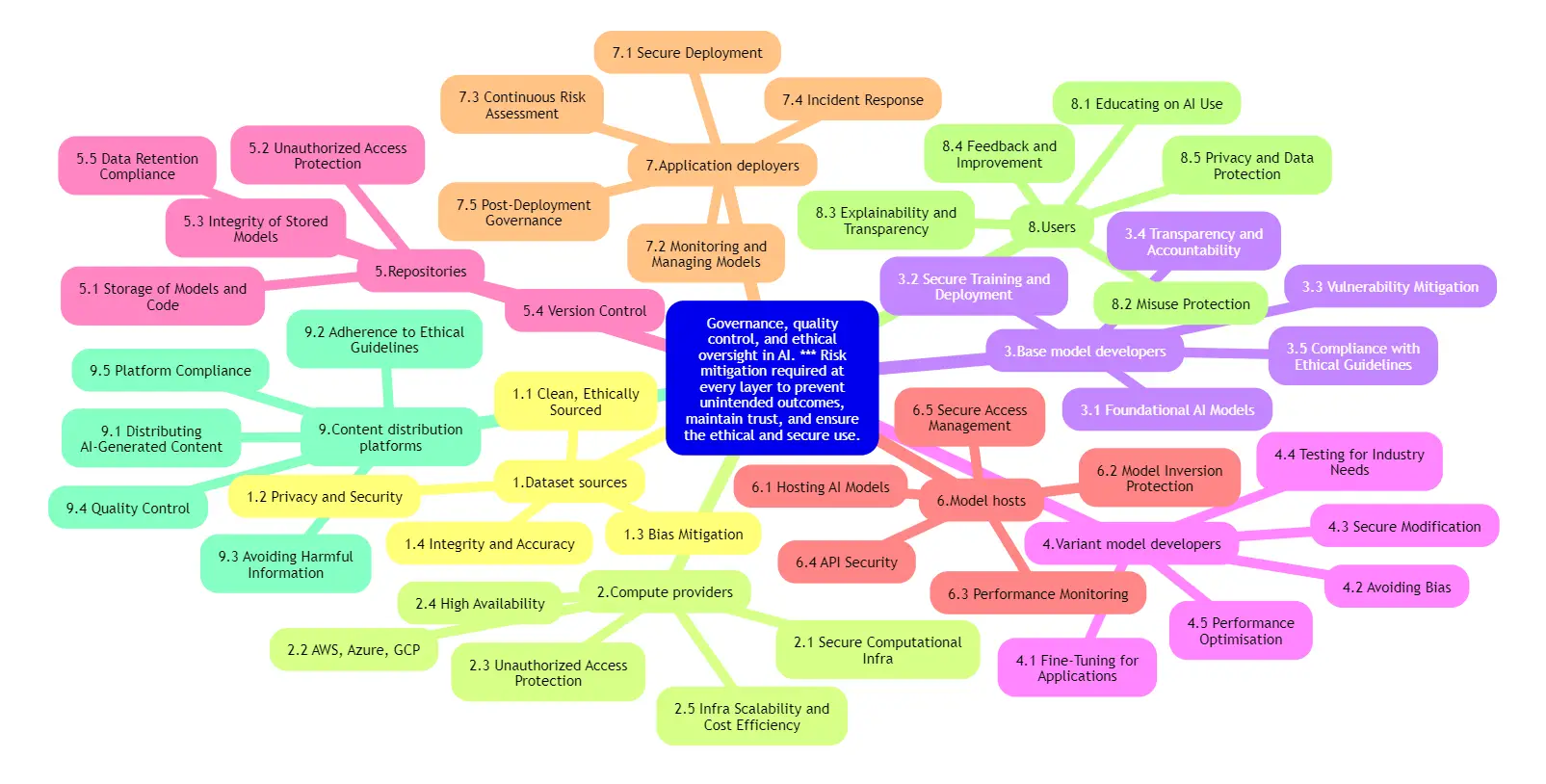
At Cintelis AI, we understand that deploying AI is more than a technological feat—it’s about managing risks at every layer to ensure the safety, fairness, and ethical use of AI.
The AI Supply Chain: Governance Beyond Cybersecurity
Much like traditional supply chains in manufacturing, the AI lifecycle consists of several interconnected players. These include data sources, developers, infrastructure providers, deployment platforms, and users, all of which contribute to the integrity of AI systems. Risk mitigation at each of these layers is critical to avoid unintended outcomes, maintain trust, and ensure compliance with ethical and legal standards. 👨💼💼
Rather than framing this process purely in terms of cybersecurity, the goal is broader. It’s about ensuring the robustness, fairness, and transparency of AI systems through consistent governance and quality oversight from start to finish. Let’s dive deeper into how this works across the key layers of the AI lifecycle:
1. Dataset Sources 📊
Data is the lifeblood of AI. Mitigating risks at this stage involves ensuring that the data is:
- Clean: Free from irrelevant or harmful information.
- Ethically sourced: Acquired in ways that respect privacy and data ownership.
- Free of bias: Avoiding skewed data that may lead to discriminatory AI behavior.
- Accurate and reliable: High-quality data is essential for robust AI models.
2. Compute Providers ☁️
These are the cloud platforms and infrastructure providers that power AI:
- AWS, Azure, GCP: Common platforms providing scalable compute resources.
- Secure infrastructure: Protecting against unauthorised access and ensuring uptime.
- High availability and scalability: Ensuring AI systems are resilient and can scale efficiently while keeping costs optimised.
3. Base Model Developers 🛠️
These are the teams or organisations responsible for developing the foundational AI models:
- Foundational models: Large language models or vision systems created by pioneers like OpenAI or Google.
- Vulnerability mitigation: Ensuring these models are trained and deployed securely, without introducing weaknesses.
- Compliance: Following strict ethical guidelines to avoid unintended consequences.
4. Variant Model Developers 🔧
These developers fine-tune base models for industry-specific needs:
- Application-specific adjustments: Tailoring models for healthcare, finance, or any other field.
- Avoiding bias: Fine-tuning without introducing new biases into the model.
- Performance optimisation: Ensuring models are efficient and accurate for their target application.
5. Repositories 💽
Repositories are where models and code are stored, such as on GitHub or Hugging Face:
- Storage and version control: Keeping track of changes and ensuring code is up-to-date.
- Unauthorized access protection: Safeguarding against tampering or theft of model data.
- Data retention compliance: Adhering to legal requirements for storing and deleting sensitive data.
6. Model Hosts 🌍
These platforms host AI models, making them accessible via APIs or direct integration:
- Model inversion protection: Guarding against attacks that attempt to reverse-engineer the model.
- API security: Ensuring secure and authorised access to hosted models.
7. Application Deployers 🚀
Responsible for deploying AI models into production environments:
- Secure deployment: Ensuring that the deployment process is airtight.
- Monitoring model behavior: Continuous oversight of the model in live environments to detect anomalies or risks.
- Incident response: Ready to act when something goes wrong, with clear governance processes in place.
8. Users 👥
The end-users or clients who interact with AI systems, either directly or indirectly:
- Educating users: Ensuring they understand the limitations and proper use of AI.
- Preventing misuse: Putting safeguards in place to prevent unethical use of AI technologies.
- Privacy and transparency: Maintaining user trust through clear communication and robust privacy protections.
9. Content Distribution Platforms 📰
These platforms distribute AI-generated content, such as automated reports or media:
- Ethical content adherence: Ensuring AI-generated content follows ethical standards.
- Quality control: Verifying the accuracy and integrity of distributed content.
- Avoiding harmful information: Preventing the spread of misinformation or harmful content generated by AI.
Why Governance, Quality Control, and Ethical Oversight Matter
AI is more than just code—it’s about making decisions that impact real lives. Ensuring the governance, quality control, and ethical oversight of AI systems helps build a future where AI works for everyone. At Cintelis AI, we focus on making AI not just smart, but fair, transparent, and secure.
Want to learn more about how we implement these principles in our AI solutions? 👉 Book a meeting with Cintelis AI for a free consultation: Booking Assistant
#AI #Governance #EthicsInAI #AIInnovation #CyberSecurity #TrustworthyAI